Deep learning models, such as those used in medical imaging to help detect disease or abnormalities, must be trained with a lot of data. However, often there isn’t enough data available to train these models, or the data is too diverse.
Ulugbek Kamilov, an associate professor of computer science and engineering and of electrical and systems engineering in the McKelvey School of Engineering at Washington University in St. Louis, along with Shirin Shoushtari, Jiaming Liu and Edward Chandler, doctoral students in his group, have developed a method to get around this common problem in image reconstruction. The team will present the results of the research this month at the International Conference on Machine Learning in Vienna, Austria.
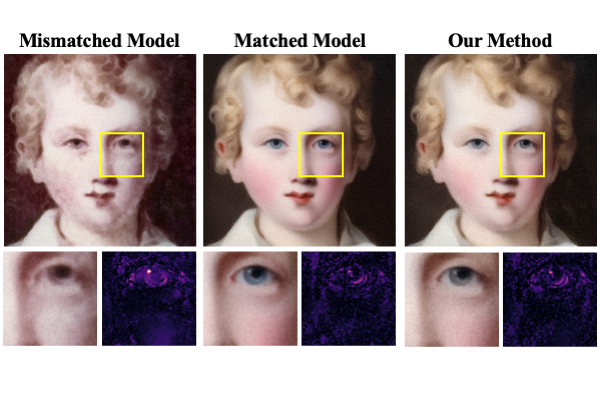
For example, MRI data used to train deep learning models could come from different vendors, hospitals, machines, patients or body parts imaged. A model trained on one type of data could introduce errors when applied on other data. To avoid those errors, the team adopted the widely used deep learning approach known as Plug-and-Play Priors, accounted for the shift in the data with which the model was trained and adapted the model to a new incoming set of data.
“With our method, it doesn’t matter if you don’t have a lot of training data,” Shoushtari said. “Our method enables to adapt deep learning models using a small set of training data, no matter what hospital, what machine or what body parts the images come from.”
Read more on the McKelvey School of Engineering website.