Artificial intelligence (AI) is showing promise in multiple medical imaging applications. Yet rigorous evaluation of these methods is important before they are introduced into clinical practice.
A multi-institutional and multiagency team led by researchers at Washington University in St. Louis is outlining a framework for objective task-based evaluation of AI-based methods and outlining the key role that physicians play in these evaluations. They also are providing techniques to conduct such evaluations, particularly in positron emission tomography (PET).
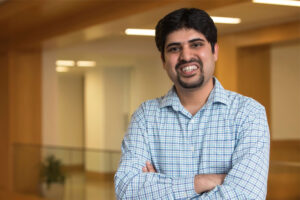
Abhinav Jha, assistant professor of biomedical engineering at the McKelvey School of Engineering, and Barry Siegel, MD, professor of radiology and of medicine at the School of Medicine, led a team that laid out such a framework in a paper published in a special October issue on AI in PET in the journal PET Clinics.
Joining the team are Kyle Myers, senior adviser at the U.S. Food and Drug Administration; Nancy Obuchowski, vice chair of quantitative health sciences and professor of medicine at the Cleveland Clinic; Babak Saboury, MD, lead radiologist (PET/MRI) and chief clinical data science officer at the National Institutes of Health (NIH); and Arman Rahmim, associate professor of radiology and of physics at the University of British Columbia (UBC) and senior scientist and provincial medical imaging physicist at BC Cancer.
“We have all of these new AI methods being developed, and while it is an exciting technology, it also is often like a black box,” said Jha, who also is assistant professor of radiology at the university’s Mallinckrodt Institute of Radiology. “This leads to multiple concerns about their applicability in medicine.”
As an example, AI is being explored to create images obtained with PET or SPECT scanners at lower doses of the radioactive tracer or shorter acquisition times.
“However, the problem is that because AI has learned from previous scans, it may produce good-looking images,” Jha said. “But studies are showing in some cases, the images contain false tumors, while at other times, the true tumor may be removed from the image. Also, studies show that the AI may work well with one data set but fail with another data set.”
In another study, an AI system that was trained with multiple dermatological images appeared more likely to classify images with rulers as malignant. In other words, the system inadvertently learned that rulers are malignant.
“Given all of these challenges, there is an important need for rigorous evaluation approaches,” Jha said. “The current approaches often evaluate how ‘good’ the images look. Studies, including those by our group, have shown that these goodness-based interpretations can be misleading and even contradict performance on clinical tasks.”
“These examples point out the need for a rigorous framework of evaluation with independent testing of AI systems using performance metrics that align with claims being made about the system,” Obuchowski added.
The framework the team proposes is based on principles from the field of objective assessment of image quality. It provides a mechanism to evaluate AI methods on tasks such as detection, quantification or both. Further, it suggests that the evaluation should model patient populations seen in the clinic, which may include different ethnicities, races, genders, body weights and ages.

“The paper by Jha and his team is a welcome contribution to the field of image science, bringing the principles of objective assessment of image quality to the practitioners of artificial intelligence who are targeting applications in nuclear medicine,” Myers said. “The medical imaging field is very much in need of robust methods to evaluate AI that are relevant and predictive of clinical performance. Further, highlighting of the role of the physician is an important aspect of this work.”
Siegel echoed Myers’ points.
“AI scientists should not work in isolation — their collaboration with physicians is very important because they can tell you what is the most important clinical task, what is the correct representative population for that task and the right way to approach the task,” Siegel said. “Clinical relevance will come from someone who is in the clinic and will be using the software.”
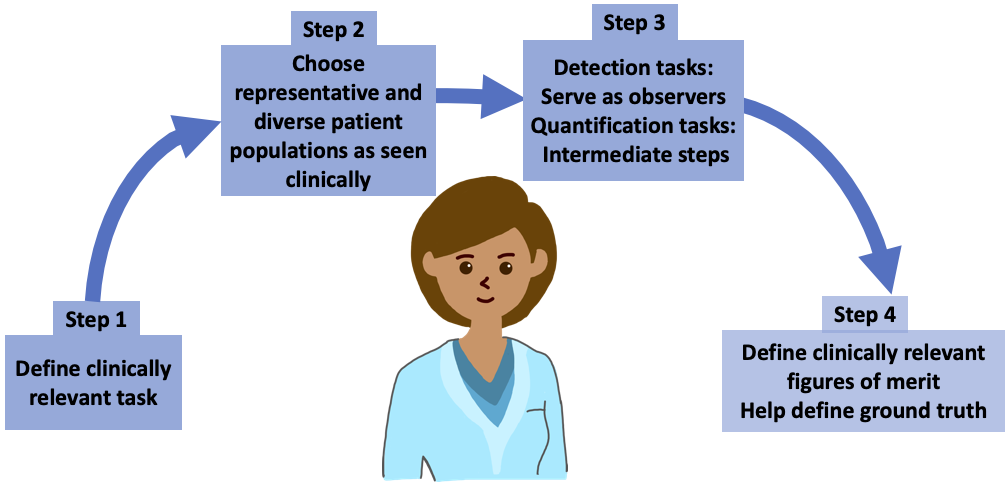
“I should remind myself all the time that medicine is the art of compassionate care using scientific wisdom and best available tools,” Saboury said. “While there are many opportunities for AI to benefit the practice of medicine, a key challenge is attention to the primacy of ‘trustworthy AI.’ This paper lays down a rigorous framework for evaluating AI methods in the direction of improving this trust.”
“We hope that this evaluation will provide trust in the clinical application of these methods, ultimately leading to improvements in quality of health care and in patient lives,” Jha said.
Jha AK, Myers KJ, Obuchowski NA, Liu Z, Rahman MA, Saboury B, Rahmim A, Siegel BA. Objective task-based evaluation of artificial intelligence-based medical imaging methods: Framework, strategies and role of the physician. PET Clinics, October 2021. https://doi.org/10.1016/j.cpet.2021.06.013
The McKelvey School of Engineering at Washington University in St. Louis promotes independent inquiry and education with an emphasis on scientific excellence, innovation and collaboration without boundaries. McKelvey Engineering has top-ranked research and graduate programs across departments, particularly in biomedical engineering, environmental engineering and computing, and has one of the most selective undergraduate programs in the country. With 140 full-time faculty, 1,387 undergraduate students, 1,448 graduate students and 21,000 living alumni, we are working to solve some of society’s greatest challenges; to prepare students to become leaders and innovate throughout their careers; and to be a catalyst of economic development for the St. Louis region and beyond.