In 2014, the Institute of Medicine (IOM), the health arm of the National Academy of Sciences, convened a committee of national experts from the fields of public health, sociology, economics, health management and policy, tobacco research, and epidemiology. The goal: provide guidance to the U.S. Food and Drug Administration (FDA) on using agent-based models (ABMs) to improve the effect of tobacco control policy on public health.
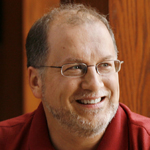
Last month, the committee, which included Douglas A. Luke, PhD, professor at the Brown School at Washington University in St. Louis and director of the Center for Public Health Systems Science, released its report titled “Assessing the Use of Agent-Based Models for Tobacco Regulation.”
The report describes the complex tobacco policy environment and provides key recommendations for policy makers and modelers to consider when developing ABMs. The committee also recommended a formal process to guide rigorous model development and evaluation.
“The Center for Tobacco Products at the FDA has been investing in the development of computation models to inform their regulatory and policy decision making,” Luke said. “This report not only affirms the importance of these modeling tools for FDA, but provides a set of model development and assessment tools that have not been available previously for policy-relevant computational models.”
Luke is a leading researcher in the areas of health behavior, organizations, policy and tobacco control. A top biostatistician and social science methodologist, he has made significant contributions to the evaluation of public health programs, tobacco control and prevention policy, and the application of new methods to community health interventions.
The new report recommends a formal process to guide rigorous ABM model development and evaluation. This new framework is designed to give modelers, funders, and policy makers guidance on how to appropriately assess the processes and outcomes of policy-relevant computational models.
According to the report, agent-based models are computational models used to examine how individual elements, or agents, of a system behave as a function of individual characteristics, the environment and interactions with each other.
Each agent interacts with other agents based on a set of rules and within an environment specified by the modeler, which leads to a set of specific aggregate outcomes, some of which may be unexpected.
With these capabilities, ABMs have the potential to provide a deeper understanding of complex behaviors and interactions of diverse individuals and their environment, and to inform policy making.