American employers increasingly rely on large datasets and computer algorithms to decide who gets interviewed, hired or promoted.
While these data algorithms can help to avoid biased human decision-making, they also risk introducing new forms of bias or reinforcing existing biases.
Pauline Kim, Daniel Noyes Kirby Professor of Law at Washington University in St. Louis, explains that when algorithms rely on inaccurate, biased or unrepresentative data, they may systematically undermine racial and ethnic minorities, women and other historically disadvantaged groups.
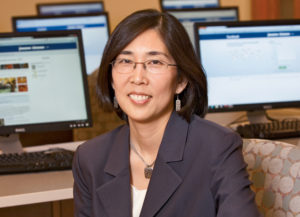
“When this happens, the result is classification bias — a term that highlights the risk that data algorithms may sort or score workers in ways that worsen inequality or disadvantage along the lines or race, sex, or other protected characteristics,” Kim said.
According to Kim, an expert on employment law, “we must fundamentally rethink how anti-discrimination laws apply in the workplace in order to address classification bias and avoid further entrenching workplace inequality.”
In a recent article, “Data-Driven Discrimination at Work,” published in the William & Mary Law Review Volume 58, Kim explains how existing employment discrimination laws must adapt to meet the challenges posed by algorithmic decision-making.
“Rote application of our existing laws will fail to address the real sources of bias when discrimination is data-driven,” Kim said.
“Because data algorithms differ significantly from traditional discriminatory practices, they require a different legal response adapted to the particular risks they raise. Focusing on classification bias suggests that anti-discrimination law should be adjusted in a number of ways when it is applied to data algorithms.”